Inproceedings3095: Unterschied zwischen den Versionen
Aus International Center for Computational Logic
Sebastian Rudolph (Diskussion | Beiträge) Keine Bearbeitungszusammenfassung |
Shima Asaadi (Diskussion | Beiträge) Keine Bearbeitungszusammenfassung |
||
Zeile 13: | Zeile 13: | ||
}} | }} | ||
{{Publikation Details | {{Publikation Details | ||
|Bild=W16-2408.pdf | |||
|Abstract=Compositional matrix-space models of language were recently proposed for the task of meaning representation of complex text structures in natural language processing. These models have been shown to be a theoretically elegant way to model compositionality in natural language. However, in practical cases, appropriate methods are required to learn such models by automatically acquiring the necessary token-to-matrix assignments. In this paper, we introduce graded matrix grammars of natural language, a variant of the matrix grammars proposed by Rudolph and Giesbrecht (2010), and show a close correspondence between this matrix-space model and weighted finite automata. We conclude that the problem of learning compositional matrix-space models can be mapped to the problem of learning weighted finite automata over the real numbers. | |Abstract=Compositional matrix-space models of language were recently proposed for the task of meaning representation of complex text structures in natural language processing. These models have been shown to be a theoretically elegant way to model compositionality in natural language. However, in practical cases, appropriate methods are required to learn such models by automatically acquiring the necessary token-to-matrix assignments. In this paper, we introduce graded matrix grammars of natural language, a variant of the matrix grammars proposed by Rudolph and Giesbrecht (2010), and show a close correspondence between this matrix-space model and weighted finite automata. We conclude that the problem of learning compositional matrix-space models can be mapped to the problem of learning weighted finite automata over the real numbers. | ||
|Projekt=QuantLA | |Projekt=QuantLA |
Version vom 29. Mai 2020, 10:03 Uhr
On the Correspondence between Compositional Matrix-Space Models of Language and Weighted Automata
Shima AsaadiShima Asaadi, Sebastian RudolphSebastian Rudolph
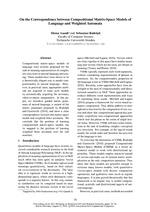
Shima Asaadi, Sebastian Rudolph
On the Correspondence between Compositional Matrix-Space Models of Language and Weighted Automata
Proceedings of the ACL Workshop on Statistical Natural Language Processing and Weighted Automata (StatFSM 2016), August 2016
On the Correspondence between Compositional Matrix-Space Models of Language and Weighted Automata
Proceedings of the ACL Workshop on Statistical Natural Language Processing and Weighted Automata (StatFSM 2016), August 2016
- KurzfassungAbstract
Compositional matrix-space models of language were recently proposed for the task of meaning representation of complex text structures in natural language processing. These models have been shown to be a theoretically elegant way to model compositionality in natural language. However, in practical cases, appropriate methods are required to learn such models by automatically acquiring the necessary token-to-matrix assignments. In this paper, we introduce graded matrix grammars of natural language, a variant of the matrix grammars proposed by Rudolph and Giesbrecht (2010), and show a close correspondence between this matrix-space model and weighted finite automata. We conclude that the problem of learning compositional matrix-space models can be mapped to the problem of learning weighted finite automata over the real numbers. - Projekt:Project: QuantLA
- Forschungsgruppe:Research Group: Computational LogicComputational Logic
@inproceedings{AR2016,
author = {Shima Asaadi and Sebastian Rudolph },
title = {On the Correspondence between Compositional Matrix-Space Models of Language andWeighted Automata},
booktitle = {Proceedings of the ACL Workshop on Statistical Natural Language Processing and Weighted Automata (StatFSM 2016)},
year = {2016},
month = {August}
}